Current News
|
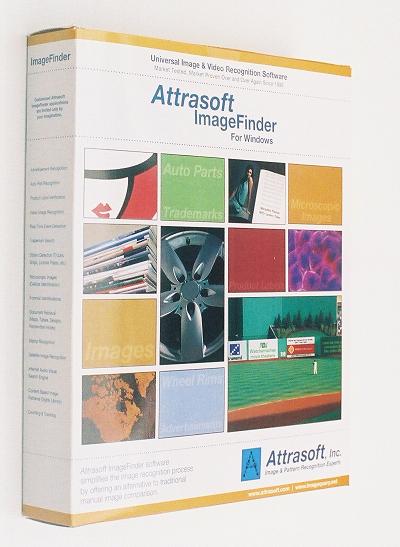
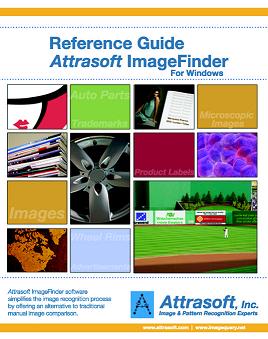
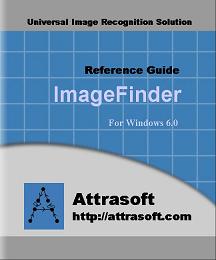
|

TransApplet 7.0, October 1, 2006
|
|
TransApplet 7.0 is release on October 1, 2006.
|
ImageFinder 7.0, September 1, 2006
|
|
ImageFinder 7.0 is release on September 1, 2006.
|
Preview: ImageFinder 7.0, June 2006
|
|
ImageFinder 7.0 will be release on August 1, 2006.
For preview, click here:
|
Publication, May 10, 2006
|
|
Attrasoft imagefinder program as a good tool for diagnosis of soft tissue
tumors, has been finaly accepted for presentation in the next IAP congress
of pathology
AUTHORS
|
NAME
|
ORGANIZATION
|
TYPE
|
Edmond Sabo
|
Department of Pathology, Rhode Island Hospital and Brown
University
|
Both
|
Ismail Zahir
|
Department of Pathology, Rhode Island Hospital
|
Author
|
James Suh
|
Department of Pathology, Rhode Island Hospital and Brown University
|
Author
|
Xu Chengen
|
Department of Pathology, Rhode Island Hospital and Brown
University
|
Author
|
Ying Liu
|
Attrasoft, Inc
|
Author
|
Murray Resnick
|
Department of Pathology, Rhode Island Hospital and Brown
University
|
Author
|
|
ABSTRACT CONTENT
|
Title (in CAPS):
|
STATISTICAL AND NEURAL PATTERN RECOGNITION METHODS FOR THE
MICROSCOPIC CLASSIFICATION OF SOFT TISSUE TUMORS: A COMPARATIVE STUDY
|
Abstract Text:
|
Background:The microscopic features of soft tissue tumors
frequently overlap. Therefore, ancillary methods are frequently required
for their diagnosis. In this preliminary study we compared two digital
technologies of pattern recognition, for their power of classification of
the following common soft tissue tumors: leiomyosarcoma, gastrointestinal
stromal tumor, fibromatosis, peripheral nerve sheath tumor, dermatofibroma
and dermatofibrosarcoma protuberance. The digital methods we used were: 1.
Computerized morphometry: a semiautomated object (tumor nuclei) oriented
method coupled with a statistical classifier (linear discriminant analysis)
and 2. Automatic digital signature formation of the images coupled with two
artificial intelligence neural network classifiers.
Design:Over 300 microscopic images representing 10 tumors in each
diagnostic category were analyzed. Images were equally divided in training
and testing sets. Computerized morphometry was done (ImageProPlus). Tumor
nuclei were evaluated for size (e.g.area), shape (e.g.ellipticity) and
texture (e.g.margination). Additional variables included nuclear
orientation and microspatial distribution. Using statistically significant
morphometrical criteria, a statistical linear discriminant function was
created in order to differentiate between tumor categories. The second
method involved preparation of image digital signatures (Image Finder-6,
Attrasoft). Classification of signature patterns was obtained using a
Boltzmann�s machine based algorithm (Attrasoft) and a backpropagation
neural network algorithm (Matlab).
Results:Computerized morphometry coupled with a statistical model
(discriminant analysis) revealed the highest classification accuracy for
differentiation between tumor categories (accuracy ranges 98% to 100% for
training sets and 75-89% for testing sets). Best differentiation was
obtained between malignant versus benign categories. The neural network
algorithms revealed lower classification accuracies (88%-100% by
back-propagation for the training sets, and 70-87% for the testing sets.
Boltzmann�s algorithm revealed similar results).
Conclusion:Computerized morphometry, a semiautomatic method, displayed
higher accuracy rates however, being much more time consuming and observer
dependent. Digital signature method coupled with artificial intelligence
presented slightly lower (but still powerful) classification rates,
presenting the advantage of being fully automated and non-observer
dependent. Overall, both methods revealed powerful classification rates for
differentiating between the soft tissue tumor categories.
|
Category:
|
Techniques
|
|
|
Trade Show April 27, 2006, Atlanta GA
|
|
Attrasoft participated The ATDC Trade Show.
|
Under Construction |
|
|
|
|
|

Copyright (c) 2006 Attrasoft. All rights reserved.
gina@attrasoft.com |